Imagine you’re applying for a loan to buy your dream house finally. You’ve saved diligently, your credit score gleams and everything seems perfect.
Then, the unthinkable happens: the AI-powered loan approval system rejects your application. Devastated and confused, you’re left with a single question: why?
This scenario, fueled by the growing presence of AI in our lives, underscores a critical need for transparency.
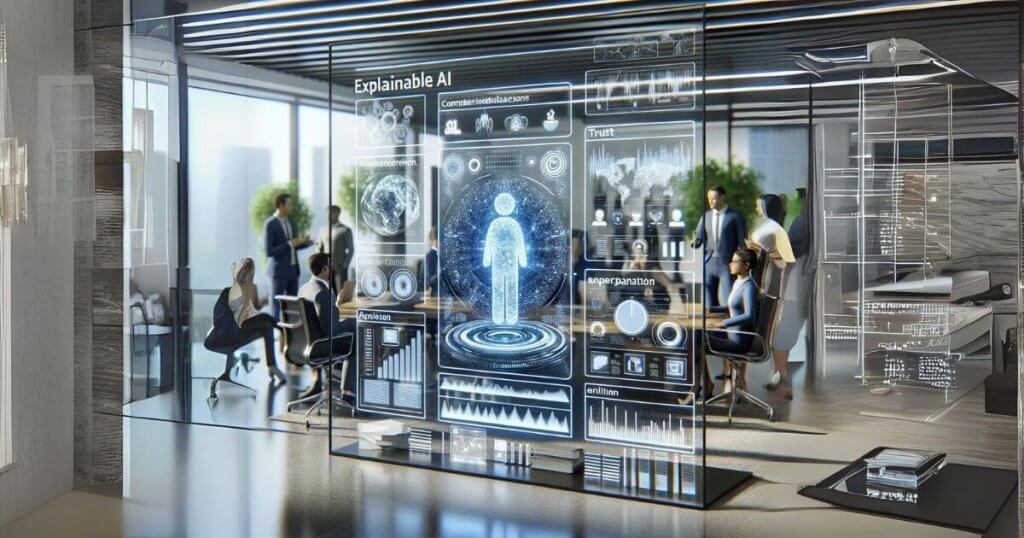
Enter Explainable AI (XAI), the superhero of the AI world, ready to demystify the “black box” and shed light on how these powerful systems make decisions.
XAI isn’t just about understanding AI for the sake of it. Trust is paramount in sectors like finance and healthcare, where AI is increasingly making critical decisions.
XAI empowers users to understand the reasoning behind an AI’s choices, fostering trust and ensuring fair and responsible implementation.
Throughout this article, we’ll delve into the fascinating world of XAI, exploring how it works, its immense benefits, and the ongoing challenges it faces.
Get ready to unlock the secrets of AI decision-making and discover how XAI is building a future where we can leverage the power of AI with confidence and clarity.
Understanding AI Decision-Making: Demystifying the Black Box
Imagine a powerful AI system analyzing your loan application. It crunches numbers, weighs factors, and delivers a verdict: approved or denied.
But what if the answer is no? It feels like peering into a black box without understanding the reasoning behind the decision. This is the essence of the black box problem in traditional AI models.
These complex algorithms often operate as opaque systems, making it difficult, if not impossible, to understand how they arrive at their conclusions.
The Rise of Explainable AI (XAI) tackles this challenge by demystifying the black box and shedding light on AI decision-making processes.
While traditional AI excels at pattern recognition and complex calculations, interpretability often takes a backseat. XAI bridges this gap by giving users insights into how AI models arrive at their predictions.
This transparency fosters trust and allows for crucial human oversight, ensuring that AI decisions are fair, unbiased, and aligned with our values.
Here’s where XAI techniques come into play. These techniques act as tools to unlock the black box, offering explanations for AI model predictions in a human-understandable way.
Here are a few prominent examples:
Feature Importance
This technique analyzes the influence of individual features (data points) on the model’s prediction.
Users can gain insights into the factors driving the AI’s decision by understanding which features have the most significant impact.
For instance, XAI using feature importance in a loan approval system might reveal that debt-to-income ratio plays the most crucial role in the decision.
This knowledge empowers lenders to explain their AI-driven decisions to loan applicants clearly and transparently.
LIME (Local Interpretable Model-agnostic Explanations)
This technique approximates the AI model’s behavior around a specific prediction.
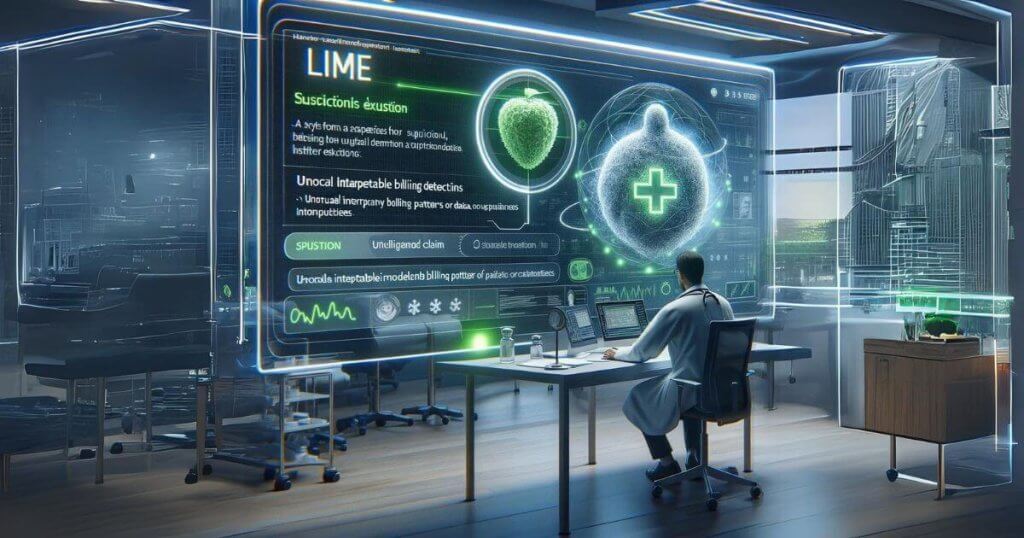
It creates a simpler, interpretable model explaining why a particular instance received a specific outcome. Imagine an AI system used for fraud detection in healthcare claims.
LIME could explain why a specific claim was flagged as suspicious, highlighting factors like unusual billing patterns or inconsistencies in the data.
This allows healthcare providers to understand the AI’s reasoning and investigate potential fraud more effectively.
SHAP (SHapley Additive exPlanations)
SHAP values go beyond simply identifying the most important features.
They explain how each feature value in a specific instance contributes to the final prediction.
Imagine a complex AI model used for loan approval, considering factors like income, credit score, and employment history.
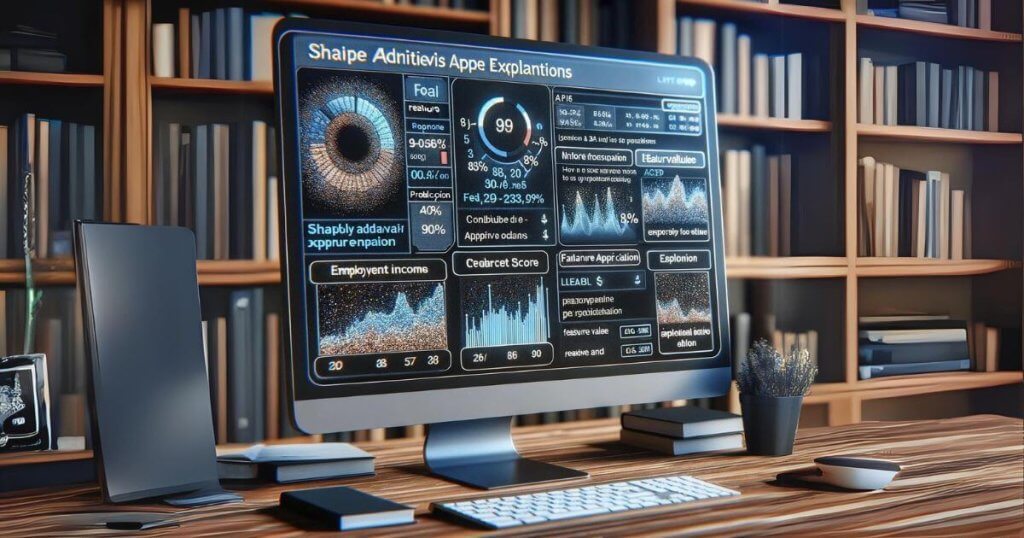
SHAP values wouldn’t just tell you that income is the most important feature; they would explain by how much a high income or a low income would influence the approval odds for a particular applicant.
Here’s a deeper dive into how SHAP values work:
- Game Theory for Explainability: SHAP borrows concepts from game theory to understand feature contributions. Imagine each feature as a player in a cooperative game, and the final prediction as the overall outcome. SHAP values then calculate a fair distribution of credit for the prediction, assigning a value to each feature based on its contribution.
- Individual Instance Explanations: Unlike some XAI techniques that provide global explanations for the entire model, SHAP values excel at explaining individual predictions. This is particularly valuable in scenarios where understanding the reasoning behind a specific AI decision is crucial. For instance, in a medical diagnosis system using AI, SHAP values could explain why a specific patient received a high-risk classification. This allows doctors to understand the AI’s reasoning, consider additional factors, and ultimately make informed medical decisions.
XAI in Action: A Case Study in Healthcare Fraud Detection
The benefits of Explainable AI extend far beyond theoretical understanding. Let’s look at a real-world example of how XAI is making a significant impact in the healthcare industry: fraud detection.
Healthcare fraud is a complex issue, costing billions of dollars annually. AI models are increasingly used to identify suspicious claims, but without transparency, trust in these systems can be limited. This is where XAI steps in.
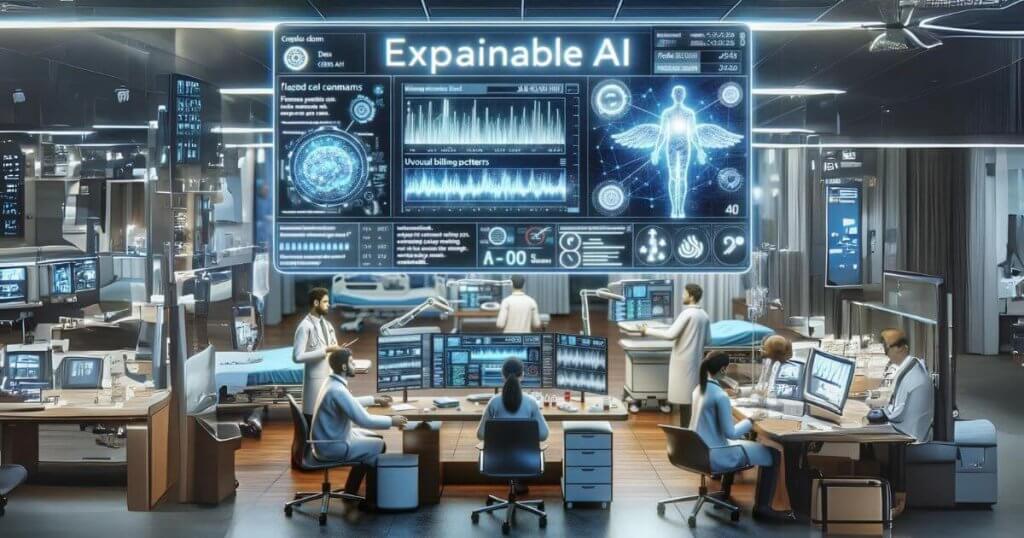
By employing XAI techniques like LIME or SHAP values, healthcare providers can gain valuable insights into how AI models flag claims as fraudulent. These insights can then be used to:
- Improve the Accuracy of AI Models: By understanding the factors contributing to false positives, healthcare providers can refine their AI models to reduce errors and improve their effectiveness in detecting actual fraud.
- Enhance Human Oversight: XAI explanations empower healthcare professionals to understand the AI’s reasoning behind a flagged claim. This allows them to leverage their expertise to investigate suspicious cases more effectively and make informed decisions.
- Build Trust and Transparency: When healthcare providers can explain why a claim was flagged for review, it fosters trust and transparency within the healthcare system. This is crucial for maintaining positive relationships with patients and ensuring the smooth operation of AI-powered fraud detection systems.
The case of healthcare fraud detection is just one example of how The Rise of Explainable AI is revolutionizing various industries.
As XAI techniques continue to evolve, we can expect even greater transparency and trust in AI systems, paving the way for a more responsible and ethical future of artificial intelligence.
The Rise of Explainable AI (XAI): Building Trust and Empowering Progress
Imagine a world where AI systems make critical decisions in areas like loan approvals or medical diagnoses.
While the power of AI is undeniable, ensuring trust in these decisions is paramount. This is where Explainable AI (XAI) emerges as a game-changer.
By shedding light on the reasoning behind AI choices, XAI unlocks a range of benefits that pave the way for a more responsible and ethical future of artificial intelligence.
One of the most significant advantages of XAI is its ability to build trust in AI systems. Traditionally, AI models have often operated as “black boxes,” making their decision-making processes opaque.
This lack of transparency can breed suspicion and hinder user acceptance. XAI bridges this gap by providing users with clear explanations for AI predictions.
- Understanding the “Why”: When users understand how an AI model arrives at a decision, it fosters a sense of control and predictability. Imagine applying for a loan and receiving a rejection alongside an explanation from the AI system highlighting specific factors that influenced the decision. This transparency empowers users to address potential shortcomings in their application or simply accept the decision with a clearer understanding.
- Transparency for Stakeholders: XAI isn’t just about user trust; it’s also crucial for stakeholders involved in AI development and deployment. Regulatory bodies and policymakers can leverage XAI to ensure AI models comply with ethical guidelines and established regulations. This fosters responsible AI development and implementation, mitigating potential risks associated with opaque AI systems.
XAI and Regulatory Compliance: A Responsible Future for AI
The increasing presence of AI in various sectors necessitates robust regulations and ethical considerations. Here’s where Explainable AI plays a vital role in ensuring responsible AI.
- Adherence to Regulations: Many industries, like finance and healthcare, operate within strict regulatory frameworks. XAI empowers developers to ensure AI models comply with these regulations by providing insights into potential biases or discriminatory practices within the model’s decision-making process. For instance, XAI techniques can help identify and mitigate biases in loan approval systems, ensuring fair and ethical treatment for all applicants.
- Aligning with Ethical Guidelines: Beyond regulations, XAI fosters the development of AI models that adhere to ethical guidelines. By enabling developers to understand how AI models arrive at decisions, XAI allows for the identification and mitigation of potential ethical concerns. For example, XAI can help identify biases in AI-powered facial recognition systems, preventing discriminatory outcomes based on race or gender.
The Rise of Explainable AI (XAI): Unlocking Potential, Addressing Challenges
While Explainable AI (XAI) offers a powerful toolkit for demystifying AI decision-making, it’s important to acknowledge the ongoing challenges in this field.
As XAI research continues to evolve, addressing these challenges will be crucial for maximizing the benefits and ensuring the responsible development of explainable AI.
One key challenge lies in the complexity of some XAI techniques.
Certain methods, particularly those designed for deep learning models, can be highly intricate and require significant technical expertise to understand and implement.
This can create a barrier for some users who may not possess the necessary background in data science or machine learning.
- Bridging the Knowledge Gap: To bridge this gap, researchers are actively developing more user-friendly XAI techniques. This includes creating intuitive visualizations and interfaces that translate complex explanations into clear and actionable insights for users with varying technical backgrounds.
- The Democratization of XAI: Furthermore, ongoing research is focused on making XAI tools more accessible and easier to integrate into the AI development process. This would empower a broader range of developers, even those without specialized XAI expertise, to build explainable AI models from the ground up.
Another challenge to consider is the potential trade-off between accuracy and explainability.
While some XAI techniques can effectively explain simple models, they may struggle with highly complex models known for achieving superior accuracy.
In some cases, making an AI model more interpretable might require sacrificing some level of accuracy.
- Finding the Right Balance: Researchers are actively exploring ways to optimize this trade-off. New XAI techniques are being developed that aim to achieve a balance between explainability and accuracy, allowing users to gain valuable insights into the decision-making process without sacrificing the overall performance of the AI model.
- Contextualizing Accuracy: It’s important to remember that accuracy is not a one-size-fits-all concept. The acceptable level of accuracy for an AI model can vary depending on the specific application. In high-stakes scenarios like healthcare diagnoses, a slight decrease in accuracy might be acceptable if it’s accompanied by a significant boost in explainability.
By acknowledging and addressing these challenges, the field of XAI can continue to mature and unlock its full potential.
The Rise of Explainable AI (XAI): Shaping a Transparent Future
The field of Explainable AI (XAI) is on a dynamic trajectory, with ongoing research and development addressing current limitations and paving the way for a future where AI operates with unparalleled transparency.
By fostering collaboration between AI researchers, developers, and policymakers, XAI has the potential to revolutionize numerous sectors and build trust in the power of artificial intelligence.
ure where AI operates with transparency and empowers us to make informed decisions alongside intelligent machines.
The Rise of Explainable AI (XAI): A Collaborative Effort
The field of XAI isn’t emerging in a vacuum. Its development is fueled by the collective efforts of various entities, each playing a crucial role in pushing the boundaries of explainability and ensuring the responsible use of AI.
Let’s explore some key players in the XAI landscape:
1. DARPA (Defense Advanced Research Projects Agency): Funding the Future of XAI
The Defense Advanced Research Projects Agency (DARPA), a U.S. government agency renowned for its groundbreaking research initiatives, has played a significant role in advancing the field of XAI. DARPA recognizes the importance of explainability in AI systems, particularly in high-stakes applications.
- Investing in Explainable AI Research: One way DARPA fosters XAI development is by funding research projects focused on creating new XAI techniques. These projects often involve collaboration between leading universities, research institutions, and private companies. DARPA’s funding helps accelerate the development of innovative XAI methods that can address current limitations and unlock the full potential of explainability.
- The DARPA Explainable AI Program: A noteworthy initiative is the DARPA Explainable AI Program, launched in 2016. This program specifically aimed to develop new XAI techniques that could explain the rationale behind AI decisions in complex scenarios. The program’s success has significantly contributed to the advancement of XAI research and paved the way for further exploration in this crucial field.
2. The Algorithmic Justice League: Advocating for Transparency and Fairness
Beyond government agencies, non-profit organizations are also playing a vital role in shaping the future of XAI. One such organization is The Algorithmic Justice League (AJL).
The AJL champions transparency and accountability in algorithmic systems, including AI.
- Raising Awareness of Bias: The AJL actively raises awareness about potential biases that can creep into AI algorithms. By highlighting these biases and their impact, the AJL emphasizes the importance of XAI in identifying and mitigating such issues. Explainable AI techniques can shed light on how AI models arrive at decisions, allowing developers to address any biases that might lead to unfair or discriminatory outcomes.
- Promoting Responsible AI Development: The AJL advocates for responsible AI development practices that prioritize fairness, transparency, and human oversight. Explainable AI aligns perfectly with these goals by ensuring users understand how AI systems work and can identify potential issues. By promoting XAI, the AJL contributes to building trust in AI and fostering its ethical development and deployment.
These are just a few examples of the diverse entities propelling the rise of XAI.
As research continues and collaboration expands, we can expect even more advancements in explainable AI, paving the way for a future where humans and AI can work together transparently and responsibly.
The Future of Explainable AI
The field of Explainable AI (XAI) is on a dynamic trajectory, with ongoing research and development addressing current limitations and paving the way for a future where AI operates with unparalleled transparency.
By fostering collaboration between AI researchers, developers, and policymakers, XAI has the potential to revolutionize numerous sectors and build trust in the power of artificial intelligence.
Advancements in XAI Research: Unveiling the Black Box Further
Researchers are actively exploring new frontiers in XAI, constantly refining existing techniques and developing novel approaches to address current limitations.
Here are some exciting advancements shaping the future of XAI:
- Explainable AI for Complex Models: One key area of focus is developing XAI techniques specifically designed for complex models, particularly deep learning models known for their high accuracy but often characterized as “black boxes.” New XAI methods are being designed to provide interpretable insights into these intricate models, ensuring we can leverage their power without sacrificing transparency.
- Counterfactual Explanations: This emerging technique goes beyond explaining a single prediction. It explores what would have happened if a specific data point had been different. Imagine an XAI system explaining a loan rejection by providing a counterfactual scenario: “If your income had been 10% higher, your loan approval odds would have increased by 20%.” This type of explanation offers valuable insights into the model’s decision-making process and allows users to understand how changes to specific factors might influence the outcome.
- Human-Centered XAI Techniques: Another exciting development is the rise of human-centered XAI techniques. These techniques prioritize user experience by creating explanations tailored to the specific needs and understanding of the user. This could involve interactive visualizations or explanations phrased in everyday language, making XAI insights more accessible to a broader audience.
The advancements mentioned above represent just a glimpse into the dynamic world of XAI research.
As researchers continue to push the boundaries, we can expect even more powerful and user-friendly XAI techniques to emerge in the coming years.
Collaboration is Key: Building Trustworthy AI Together
The responsible development and deployment of XAI necessitates collaboration across various domains. Here’s how different stakeholders can contribute to the future of XAI:
- AI Researchers and Developers: Researchers play a crucial role in pushing the boundaries of XAI research by developing new techniques and refining existing ones. Developers, on the other hand, can integrate XAI tools into their workflows, ensuring explainability is prioritized throughout the AI development lifecycle. This collaboration between researchers and developers is fundamental for ensuring XAI becomes a standard practice in AI development.
- Policymakers and Regulators: Policymakers have a critical role in establishing clear guidelines and regulations for explainable AI. This fosters trust in AI systems and encourages responsible development practices within the industry. Regulations could, for instance, mandate the use of XAI techniques in specific high-risk applications, such as healthcare or finance.
- Industry and End-Users: Industries that leverage AI can play a vital role by actively seeking out and implementing XAI solutions. This sends a strong signal to developers about the importance of explainability and creates a market demand for XAI tools. End-users, by advocating for transparency in AI systems, can also contribute to shaping the future of XAI.
By working together, these stakeholders can ensure XAI reaches its full potential, shaping a future where AI operates with transparency and empowers us to make informed decisions alongside intelligent machines.
Benefits and Challenges of Explainable AI (XAI)
Here are the benefits and challenges of Explainable AI (XAI)
Benefit of XAI | Description | Example |
Builds Trust | XAI allows users to understand the reasoning behind AI decisions, fostering trust and acceptance. | In a loan approval system, XAI can explain why a loan was rejected, helping the applicant understand areas for improvement. |
Improves Model Development | XAI helps identify potential biases or errors within AI models, allowing for refinement and better performance. | By analyzing XAI explanations, developers can identify factors leading to unfair loan rejections and adjust the model for fairer outcomes. |
Regulatory Compliance | XAI aids in ensuring AI models comply with regulations and ethical guidelines in various industries. | XAI can help financial institutions demonstrate how their loan approval models comply with anti-discrimination laws. |
Challenges of XAI | Description | Example |
Complexity of Techniques | Some XAI techniques, especially for complex models, can be intricate and require technical expertise to understand. | Understanding how a deep learning model arrives at a decision might require advanced knowledge of machine learning. |
Trade-Off Between Accuracy and Explainability | In some cases, making an AI model more interpretable might require sacrificing some level of accuracy. | A highly accurate facial recognition system might become slightly less accurate if modified to be more explainable. |
Frequently Asked Questions
How does Explainable AI work?
Explainable AI (XAI) uses various techniques to explain how AI models arrive at decisions. One common technique, feature importance, highlights which factors (features) in the data most influenced the model’s prediction. For instance, an XAI explanation for a loan approval system might reveal that income was the most important feature, but it would also detail how a high income or low income would affect the approval odds for a specific applicant.
What are the benefits of Explainable AI?
XAI offers several benefits. It builds trust in AI systems by allowing users to understand the reasoning behind decisions. This transparency is crucial for areas like healthcare or finance. Additionally, XAI helps improve model development by identifying potential biases or errors within the AI model. By understanding these issues, developers can refine the model for better performance.
Is Explainable AI necessary?
AI models that lack transparency, often called “black boxes,” can be risky. Without explanations for their decisions, it’s difficult to trust these models or identify potential biases that might lead to unfair outcomes. XAI helps mitigate these risks by providing much-needed clarity and fostering responsible AI development.
Conclusion: Unveiling the Black Box: The Rise of Explainable AI
As AI continues to permeate our lives, the need for transparency and trust in these powerful systems becomes paramount.
This is where Explainable AI (XAI) emerges as a game-changer. By shedding light on how AI models arrive at decisions, XAI unlocks a multitude of benefits.
From fostering trust in loan approval processes to improving the fairness of healthcare fraud detection, XAI has the potential to revolutionize various sectors and ensure responsible AI development.
XAI empowers users to understand the reasoning behind AI decisions, fostering a sense of control and predictability.
This transparency is crucial for building trust in AI, especially in high-stakes domains like healthcare or finance. Furthermore, XAI aids in developing better AI models by identifying potential biases or errors, ultimately leading to more accurate and fair AI systems.
The future of XAI is bright, with ongoing research addressing current limitations and developing more user-friendly techniques.
Collaboration between researchers, developers, policymakers, and end-users is key to unlocking the full potential of XAI and shaping a future where AI operates with transparency and empowers us to make informed decisions alongside intelligent machines.
Ready to delve deeper into the exciting world of XAI? Explore the following resources to learn more about XAI applications across various industries and their transformative potential for society:
- A related article on our website: The Future of AI Innovation: Trends and Challenges explores the broader landscape of AI advancements, where XAI plays a vital role in ensuring responsible and trustworthy AI development.
- Research papers and articles from leading AI organizations can provide in-depth insights into the latest XAI techniques and advancements.
Remember, as AI continues to evolve, XAI will be critical for ensuring transparency, fairness, and trust in these powerful tools.
By staying informed and advocating for responsible AI development, we can all play a role in shaping a future where AI benefits everyone.
Unleash the full potential of AI in your organization! Our comprehensive guide explores the latest advancements in AI innovation and equips you with the knowledge to make informed decisions. Learn more: The Future of AI Innovation: Trends & Challenges